\\n\\nIn the rapid tide of artificial intelligence development, accurately assessing the capabilities of AI models has become a key issue in industrial progress. On the journey to explore the boundaries of AI capabilities, researchers have come to deeply recognize the limitations of existing evaluation systems. This has spurred scholars to collaborate with top industry research institutions to jointly break through the established paradigms of AI evaluation. Against this backdrop, the milestone project SuperGPQA has emerged.\\n\\n\u003Cdiv class=\\\"img-wrap has-caption center\\\" style=\\\"width: 100%; position: relative; margin-bottom: 62px\\\">\\n \u003Cimg src=\\\"https://global-blog.oss-ap-southeast-1.aliyuncs.com/abaka/20250309-2077AI/ScalingLLM.png\\\" alt=\\\"SuperGPQA: Scaling LLM Evaluation across 285 Graduate Disciplines\\\" style=\\\"width: 100%; max-height: 60vh; object-fit: contain; background: #141414; border-radius: 8px\\\">\u003C/img>\\n \u003Cp class=\\\"img-text\\\" style=\\\"position: absolute; top: calc(100% + 16px); left: 0; right: 0;text-align: center; overflow: hidden; white-space: nowrap; text-overflow: ellipsis; line-height: 22px; color: #A1A1A1; font-size: 14px\\\">\\n SuperGPQA: Scaling LLM Evaluation across 285 Graduate Disciplines\\n \u003C/p>\\n\u003C/div>\\n\\n## 1. Insights on the Frontier: The Real Challenges of AI Evaluation\\n\\nWith large language models like GPT-4 and Claude demonstrating capabilities that approach or even surpass human levels in mainstream academic fields, accurately assessing the true proficiency of AI in a broader range of specialized areas has become an urgent challenge. Existing evaluation benchmarks, such as MMLU and GPQA, suffer from severe imbalances in subject coverage—long-tail disciplines like light industry, agriculture, and service science have a coverage rate of less than 5%, and the discriminative power of evaluation questions is gradually diminishing.\\n\\nIn response to this real challenge, 2077AI, in collaboration with top research institutions, spent six months developing the SuperGPQA project. It has, for the first time, achieved a benchmark for AI evaluation covering 285 graduate-level subjects.\\n\\n\u003Cdiv class=\\\"img-wrap has-caption center\\\" style=\\\"width: 100%; position: relative; margin-bottom: 62px\\\">\\n \u003Cimg src=\\\"https://global-blog.oss-ap-southeast-1.aliyuncs.com/abaka/20250309-2077AI/BenchmarkComparison.png\\\" alt=\\\"Comparison of benchmarks for different models\\\" style=\\\"width: 100%; max-height: 60vh; object-fit: contain; background: #141414; border-radius: 8px\\\">\u003C/img>\\n \u003Cp class=\\\"img-text\\\" style=\\\"position: absolute; top: calc(100% + 16px); left: 0; right: 0;text-align: center; overflow: hidden; white-space: nowrap; text-overflow: ellipsis; line-height: 22px; color: #A1A1A1; font-size: 14px\\\">\\n Comparison of benchmarks for different models\\n \u003C/p>\\n\u003C/div>\\n\\n## 2. Breakthrough Innovation: Restructuring the Evaluation Paradigm\\n\\nSuperGPQA has achieved breakthrough innovations in both scale and depth. The project has constructed a vast knowledge system comprising 26,529 specialized questions, far exceeding the 448 questions in GPQA and the 12,032 questions in MMLU-Pro. In terms of subject coverage, SuperGPQA spans 13 major categories, 72 first-level disciplines, and 285 second-level disciplines, achieving a comprehensive mapping of the human knowledge system. Each question is equipped with an average of 9.67 options, significantly higher than the traditional four-option format, which greatly increases the challenge of the evaluation. Notably, 42.33% of the selected questions require mathematical calculations or formal reasoning. This design ensures the evaluative distinction and depth of the assessment.\\n\\n\u003Cdiv class=\\\"img-wrap has-caption center\\\" style=\\\"width: 100%; position: relative; margin-bottom: 62px\\\">\\n \u003Cimg src=\\\"https://global-blog.oss-ap-southeast-1.aliyuncs.com/abaka/20250309-2077AI/SuperGPQA.png\\\" alt=\\\"The data collection process of SuperGPQA\\\" style=\\\"width: 100%; max-height: 60vh; object-fit: contain; background: #141414; border-radius: 8px\\\">\u003C/img>\\n \u003Cp class=\\\"img-text\\\" style=\\\"position: absolute; top: calc(100% + 16px); left: 0; right: 0;text-align: center; overflow: hidden; white-space: nowrap; text-overflow: ellipsis; line-height: 22px; color: #A1A1A1; font-size: 14px\\\">\\n The data collection process of SuperGPQA\\n \u003C/p>\\n\u003C/div>\\n\\n### 2.1 Technical Highlights: Interdisciplinary Semantic Analysis\\n\\nThrough t-SNE visualization analysis, the research team discovered that SuperGPQA exhibits a unique interdisciplinary clustering pattern in the semantic space. Questions from engineering and science demonstrate high semantic similarity, while those from the humanities maintain their distinct knowledge centers. The clustering of different disciplines achieves a complete mapping of the diverse human knowledge system. This distribution characteristic also validates the scientific nature and comprehensiveness of the evaluation dataset.\\n\\n\u003Cdiv class=\\\"img-wrap has-caption center\\\" style=\\\"width: 100%; position: relative; margin-bottom: 62px\\\">\\n \u003Cimg src=\\\"https://global-blog.oss-ap-southeast-1.aliyuncs.com/abaka/20250309-2077AI/Visualization.png\\\" alt=\\\"For the visualization of subject problem sampling\\\" style=\\\"width: 100%; max-height: 60vh; object-fit: contain; background: #141414; border-radius: 8px\\\">\u003C/img>\\n \u003Cp class=\\\"img-text\\\" style=\\\"position: absolute; top: calc(100% + 16px); left: 0; right: 0;text-align: center; overflow: hidden; white-space: nowrap; text-overflow: ellipsis; line-height: 22px; color: #A1A1A1; font-size: 14px\\\">\\n For the visualization of subject problem sampling\\n \u003C/p>\\n\u003C/div>\\n\\n\\n### 2.2 Methodological Innovation: Three-Stage Quality Control\\n\\nTo ensure the reliability of the evaluation, the project team has designed a rigorous three-stage quality control mechanism.\\n\\nIn the source screening phase, the SuperGPQA team abandoned traditional crowdsourcing methods and instead selected original questions from textbooks and authoritative materials by a team of experts.\\n\\nIn the standardization transcription phase, a professional team normalized the academic language and unified the format of all questions, ensuring that the average length of each question was maintained at 58.42 characters and guaranteeing the consistency and comparability of the options.\\n\\nIn the quality inspection phase, the research team integrated automated rule checks, cross-validation by multiple models, and in-depth expert reviews to build a robust quality assurance system.\\n\\n\u003Cdiv class=\\\"img-wrap has-caption center\\\" style=\\\"width: 100%; position: relative; margin-bottom: 62px\\\">\\n \u003Cimg src=\\\"https://global-blog.oss-ap-southeast-1.aliyuncs.com/abaka/20250309-2077AI/RewritingProcess.png\\\" alt=\\\"The complex rewriting process of correct and incorrect judgment questions in SuperGPQA\\\" style=\\\"width: 100%; max-height: 60vh; object-fit: contain; background: #141414; border-radius: 8px\\\">\u003C/img>\\n \u003Cp class=\\\"img-text\\\" style=\\\"position: absolute; top: calc(100% + 16px); left: 0; right: 0;text-align: center; overflow: hidden; white-space: nowrap; text-overflow: ellipsis; line-height: 22px; color: #A1A1A1; font-size: 14px\\\">\\n The complex rewriting process of correct and incorrect judgment questions in SuperGPQA\\n \u003C/p>\\n\u003C/div>\\n\\n## 3. Key Findings: Revealing the Boundaries of AI Capabilities\\n\\nThrough systematic evaluation of 51 mainstream models, the research team has made a series of important discoveries.\\n\\nUnder the evaluation criteria of SuperGPQA, even the best-performing DeepSeek-R1 model only achieved an accuracy rate of 61.82% in answering interdisciplinary questions. This result clearly reveals the significant gap that exists between current AI and general artificial intelligence. Experimental data indicates that instruction fine-tuning has a significant positive impact on model performance. For example, the accuracy rate of the instruction fine-tuned version of DeepSeek-V3 (47.40%) is far higher than that of its base version (32.14%).\\n\\n\u003Cdiv class=\\\"img-wrap has-caption center\\\" style=\\\"width: 100%; position: relative; margin-bottom: 62px\\\">\\n \u003Cimg src=\\\"https://global-blog.oss-ap-southeast-1.aliyuncs.com/abaka/20250309-2077AI/SuperGPQAStandard.png\\\" alt=\\\"The performance of different models under the SuperGPQA standard\\\" style=\\\"width: 100%; max-height: 60vh; object-fit: contain; background: #141414; border-radius: 8px\\\">\u003C/img>\\n \u003Cp class=\\\"img-text\\\" style=\\\"position: absolute; top: calc(100% + 16px); left: 0; right: 0;text-align: center; overflow: hidden; white-space: nowrap; text-overflow: ellipsis; line-height: 22px; color: #A1A1A1; font-size: 14px\\\">\\n The performance of different models under the SuperGPQA standard\\n \u003C/p>\\n\u003C/div>\\n\\nDuring the in-depth analysis, the research team observed a clear correlation between model scale and performance equilibrium. DeepSeek-R1 demonstrated stable performance across questions of varying difficulty levels, with an accuracy rate of 63.59% on easy questions, 63.63% on medium-difficulty questions, and 56.87% on difficult ones. Additionally, the performance improvement brought about by model version iteration was also significant. For example, the accuracy rate of the GPT-4o series steadily increased from 39.76% to 44.40% with each version update.\\n\\n## 4. Future Outlook\\n\\nThe open-source release of SuperGPQA not only fills an important gap in the field of AI evaluation but also pioneers a new research paradigm. This breakthrough provides the academic and industrial communities with a reliable \\\"compass,\\\" guiding the direction of AI technology development. As a core participant in the SuperGPQA project, 2077AI, together with the project team, has jointly planned the future development direction of the evaluation system. The research team will continue to expand the dimensions of evaluation, introduce more refined assessment criteria in specialized fields, develop dynamic difficulty adjustment mechanisms, and build cross-lingual evaluation capabilities. On the methodological front, the project team is committed to optimizing human-machine collaborative evaluation mechanisms, developing adaptive question generation technologies, and establishing a more detailed capability classification system. Meanwhile, the SuperGPQA team will also vigorously promote the open-source sharing of evaluation standards, establish a global collaborative research network, and foster in-depth integration of industry, academia, and research.\\n\",\"headings\":[{\"level\":2,\"text\":\"Introduction\",\"children\":[]},{\"level\":2,\"text\":\"1. Insights on the Frontier: The Real Challenges of AI Evaluation\",\"children\":[]},{\"level\":2,\"text\":\"2. Breakthrough Innovation: Restructuring the Evaluation Paradigm\",\"children\":[{\"level\":3,\"text\":\"2.1 Technical Highlights: Interdisciplinary Semantic Analysis\",\"children\":[]},{\"level\":3,\"text\":\"2.2 Methodological Innovation: Three-Stage Quality Control\",\"children\":[]}]},{\"level\":2,\"text\":\"3. Key Findings: Revealing the Boundaries of AI Capabilities\",\"children\":[]},{\"level\":2,\"text\":\"4. Future Outlook\",\"children\":[]}]},{\"slug\":\"matrix-dataset\",\"link\":\"/blog/matrix-dataset\",\"title\":\"Matrix Dataset: A Revolutionary Bilingual AI Pre-training Corpus\",\"desc\":\"A Revolutionary Bilingual AI Pre-training Corpus\",\"bannerImg\":\"/bg1.png\",\"date\":\"2024-09-12\",\"content\":\"\\n# Matrix Dataset: A Revolutionary Bilingual AI Pre-training Corpus\\n\\n2077AI Foundation proudly announces our open-source project — the Matrix Dataset. As pioneers in AI data standardization and advancement, we are committed to unlocking AI's potential through high-quality data, accelerating AI development, and nurturing an efficient, thriving AI data ecosystem. The Matrix Dataset is a crucial component of this vision.\\n\\n\u003Cdiv class=\\\"img-wrap has-caption\\\" style=\\\"width: 100%\\\">\\n \u003Cimg src=\\\"https://global-blog.oss-ap-southeast-1.aliyuncs.com/2077ai/20240912/MatrixDataset/1.png\\\" alt=\\\"Statistics of the Matrix Pile Data Distribution: The inner pie chart represents the languagedistribution, while the outer loop indicates the proportion of meta-categories in the corpus.\\\" style=\\\"width: 100%\\\">\\n \u003Cp style=\\\"text-align: center\\\">Statistics of the Matrix Pile Data Distribution: The inner pie chart represents the languagedistribution, while the outer loop indicates the proportion of meta-categories in the corpus.\u003C/p>\\n\u003C/div>\\n\\n**What is the Matrix Dataset?**\\n\\nMatrix is a high-quality bilingual (Chinese-English) dataset containing 4690 billion tokens. It stands as the only large-scale bilingual pre-training dataset in the open-source community that can be used directly without additional calibration or validation. Its uniqueness lies not only in its scale but also in its meticulously designed data processing pipeline and diverse data sources, ensuring both high quality and broad applicability.\\n\\n**Diversity of Data Sources**\\n\\nWe built this corpus from the ground up, encompassing a wide range of topics, primarily including:\\n\\n1. Integration of existing open-source pre-training data\\n2. Additional Chinese, mathematics, and science exam data, as well as Wikipedia data collected from Common Crawl (CC)\\n3. PDF documents converted to text via OCR technology and incorporated into the dataset\\n\\n\u003Cdiv class=\\\"img-wrap has-caption\\\" style=\\\"width: 100%\\\">\\n \u003Cimg src=\\\"https://global-blog.oss-ap-southeast-1.aliyuncs.com/2077ai/20240912/MatrixDataset/image.png\\\" alt=\\\"The composition sources of re-processed English web subset. The proportion denotes dividing the size of the current dataset by the total size of the whole dataset.\\\" style=\\\"width: 100%\\\">\\n \u003Cp style=\\\"text-align: center\\\">The composition sources of re-processed English web subset. The proportion denotes dividing the size of the current dataset by the total size of the whole dataset.\u003C/p>\\n\u003C/div>\\n\\n\u003Cdiv class=\\\"img-wrap has-caption\\\" style=\\\"width: 100%\\\">\\n \u003Cimg src=\\\"https://global-blog.oss-ap-southeast-1.aliyuncs.com/2077ai/20240912/MatrixDataset/image1.png\\\" alt=\\\"The composition sources of the Chinese web subset.\\\" style=\\\"width: 100%\\\">\\n \u003Cp style=\\\"text-align: center\\\">The composition sources of the Chinese web subset.\u003C/p>\\n\u003C/div>\\n\\n**Meticulous Data Processing**\\n\\nThe excellence of the Matrix Dataset stems from our carefully designed data processing pipeline:\\n\\n1. High-standard cleaning and filtering thresholds\\n2. Strict deduplication strategies, including substring-level deduplication\\n3. Post-calibration processing for OCR data\\n\\nThrough this rigorous processing workflow, we retained only a small portion of the original data: 4% of existing corpora and 19% of crawled corpora. Each data cleaning rule was repeatedly sampled and confirmed by team members, ensuring extremely high-quality standards for both Chinese and English data.\\n\\n\u003Cdiv class=\\\"img-wrap has-caption\\\" style=\\\"width: 100%\\\">\\n \u003Cimg src=\\\"https://global-blog.oss-ap-southeast-1.aliyuncs.com/2077ai/20240912/MatrixDataset/image2.png\\\" alt=\\\"left: Re-processing retention rates; right: Processing retention rates, Funnel Diagram for the two main data pipelines. The darker part of each row represents the retention proportion for each processing step and the lighter one for the filtered corpora.\\\" style=\\\"width: 100%\\\">\\n \u003Cp style=\\\"text-align: center\\\">left: Re-processing retention rates; right: Processing retention rates, Funnel Diagram for the two main data pipelines. The darker part of each row represents the retention proportion for each processing step and the lighter one for the filtered corpora.\u003C/p>\\n\u003C/div>\\n\\n**Optimized Data Composition**\\n\\nTo maximize pre-training effectiveness, we adopted a heuristic data composition strategy:\\n\\n1. Pre-training is divided into two stages, with CC data included in the first stage and removed in the second\\n2. Manual increase in the proportion of code, books, and document-type data\\n3. Integration of deepseek-math and OCR pipelines, significantly enhancing training performance\\n\\n\u003Cdiv class=\\\"img-wrap has-caption\\\" style=\\\"width: 100%\\\">\\n \u003Cimg src=\\\"https://global-blog.oss-ap-southeast-1.aliyuncs.com/2077ai/20240912/MatrixDataset/image3.png\\\" alt=\\\"Details of Heuristic Rules for English Texts\\\" style=\\\"width: 100%\\\">\\n \u003Cp style=\\\"text-align: center\\\">Details of Heuristic Rules for English Texts\u003C/p>\\n\u003C/div>\\n\\n\u003Cdiv class=\\\"img-wrap has-caption\\\" style=\\\"width: 100%\\\">\\n \u003Cimg src=\\\"https://global-blog.oss-ap-southeast-1.aliyuncs.com/2077ai/20240912/MatrixDataset/image4.png\\\" alt=\\\"Details of Heuristic Rules for Chinese Texts.\\\" style=\\\"width: 100%\\\">\\n \u003Cp style=\\\"text-align: center\\\">Details of Heuristic Rules for Chinese Texts.\u003C/p>\\n\u003C/div>\\n\\n\u003Cdiv class=\\\"img-wrap has-caption\\\" style=\\\"width: 100%\\\">\\n \u003Cimg src=\\\"https://global-blog.oss-ap-southeast-1.aliyuncs.com/2077ai/20240912/MatrixDataset/image5.png\\\" alt=\\\"The document conversion framework is composed of various sub-models for different parts.\\\" style=\\\"width: 100%\\\">\\n \u003Cp style=\\\"text-align: center\\\">The document conversion framework is composed of various sub-models for different parts.\u003C/p>\\n\u003C/div>\\n\\n**Commitment to Open Source**\\n\\nAdhering to the principles of openness and sharing, we have not only open-sourced all the data but also made public the code for all processing pipelines, including filtering, deduplication, document conversion, and all other procedures. This allows researchers and developers to delve into every step of data processing and even adjust and optimize according to their needs.\\n\\n**Join Us in Shaping the Future of AI**\\n\\nThe launch of the Matrix Dataset marks a new milestone in AI pre-training data. We cordially invite AI researchers and developers worldwide to join us in leveraging this rich resource, pushing the boundaries of AI technology, and collectively shaping the future of AI.\\n\",\"headings\":[]},{\"slug\":\"OmniHD-Scenes\",\"link\":\"/OmniHD-Scenes\",\"title\":\"OmniHD-Scenes: A Next-Generation Multimodal Dataset for Autonomous Driving\",\"desc\":\"The 2077AI Foundation has released a new generation of datasets for the autonomous driving. These datasets provide large-scale, more modern, and more realistic data that offer perspectives not previously available.\",\"bannerImg\":\"/bg2.png\",\"date\":\"2025-03-25\",\"content\":\"\\n# OmniHD-Scenes: A Next-Generation Multimodal Dataset for Autonomous Driving\\n\\nThe 2077AI Foundation, in collaboration with Automotive Studies, Automotive Studies, Tongji University, CAERI (China Automotive Engineering Research Institute), and Geometrical-PAL, has released a new generation of datasets for the autonomous driving. These datasets provide large-scale, more modern, and more realistic data that offer perspectives not previously available. They support various downstream tasks in autonomous driving research and development.\\n\\n## Overview\\n\\n\u003Cdiv class=\\\"img-wrap has-caption center\\\" style=\\\"width: 100%; position: relative; margin-bottom: 62px\\\">\\n \u003Cimg src=\\\"https://global-blog.oss-ap-southeast-1.aliyuncs.com/2077ai/20250325/logo2.jpg\\\" alt=\\\"\\\" style=\\\"width: 100%; max-height: 60vh; object-fit: contain; background: #141414; border-radius: 8px\\\">\u003C/img>\\n \u003Cp class=\\\"img-text\\\" style=\\\"position: absolute; top: calc(100% + 16px); left: 0; right: 0;text-align: center; overflow: hidden; white-space: nowrap; text-overflow: ellipsis; line-height: 22px; color: #A1A1A1; font-size: 14px\\\">\\n \u003C/p>\\n\u003C/div>\\n\\n## Abstract\\n\\nThe OmniHD-Scenes dataset combines data from 128-beam LiDAR, six cameras, and six 4D imaging radar systems to achieve full environmental perception. The dataset comprises 1501 clips, each approximately 30s long, totaling more than 450K synchronized frames and more than 5.85 million synchronized sensor data points. We also propose a novel 4D annotation pipeline. To date, we have annotated 200 clips with more than 514K precise 3D bounding boxes. These clips also include semantic segmentation annotations for static scene elements. Additionally, we introduce a novel automated pipeline for generation of the dense occupancy ground truth, which effectively leverages information from non-key frames. Alongside the proposed dataset, we establish comprehensive evaluation metrics, baseline models, and benchmarks for 3D detection and semantic occupancy prediction. These benchmarks utilize surround-view cameras and 4D imaging radar to explore cost-effective sensor solutions for autonomous driving applications.\\n\\n\u003Cdiv style=\\\"display: flex; justify-content: space-between; max-width: 100%; overflow: hidden\\\">\\n \u003Cdiv class=\\\"img-wrap has-caption\\\" style=\\\"flex: 1; max-width: 49%; max-height: 60vh; position: relative; margin-bottom: 62px\\\">\\n \u003Cimg src=\\\"https://global-blog.oss-ap-southeast-1.aliyuncs.com/abaka/20250325-OmniHD-Scenes/Sensor-Deployment-Architecture-Diagram.png\\\" alt=\\\"First Image\\\" style=\\\"width: 100%; height: 80%; object-fit: contain; background: #141414; border-radius: 8px\\\"/>\\n \u003Cp class=\\\"img-text\\\" style=\\\"position: absolute; top: calc(100% + 16px); left: 0; right: 0;text-align: center; overflow: hidden; white-space: nowrap; text-overflow: ellipsis; line-height: 22px; color: #A1A1A1; font-size: 14px\\\">\\n Sensor Deployment Architecture Diagram\\n \u003C/p>\\n \u003C/div>\\n \u003Cdiv class=\\\"img-wrap has-caption\\\" style=\\\"flex: 1; max-width: 49%; max-height: 60vh; position: relative; margin-bottom: 62px\\\">\\n \u003Cimg src=\\\"https://global-blog.oss-ap-southeast-1.aliyuncs.com/abaka/20250325-OmniHD-Scenes/Sensor-Coordinate-System.png\\\" alt=\\\"Second Image\\\"style=\\\"width: 100%; height: 80%; object-fit: contain; background: #141414; border-radius: 8px\\\"/>\\n \u003Cp class=\\\"img-text\\\" style=\\\"position: absolute; top: calc(100% + 16px); left: 0; right: 0;text-align: center; overflow: hidden; white-space: nowrap; text-overflow: ellipsis; line-height: 22px; color: #A1A1A1; font-size: 14px\\\">\\n Sensor Coordinate System\\n \u003C/p>\\n \u003C/div>\\n\u003C/div>\\n\\nThe contributions of this study can be summarized as follows.\\n\\n1. Novel Dataset: Introduces OmniHD-Scenes, the first multimodal dataset with 4D radar point clouds for 3D object detection, multi-object tracking, and occupancy prediction.\\n2. Extensive Data: Features 1501 clips (450K+ frames, 5.85M data points) covering diverse urban driving scenarios, including challenging conditions like rain and night.\\n3. Advanced Annotation: A novel 4D annotation pipeline leverages temporal data for efficient and accurate 3D bounding box and semantic segmentation annotation.\\n4. Comprehensive Benchmarks: Establishes benchmarks for 3D object detection and occupancy prediction, including baseline models using various sensor modalities.\\n\\n## Overview\\n\\n\u003Cdiv class=\\\"img-wrap has-caption center\\\" style=\\\"width: 100%; position: relative; margin-bottom: 62px\\\">\\n \u003Cimg src=\\\"https://global-blog.oss-ap-southeast-1.aliyuncs.com/abaka/20250325-OmniHD-Scenes/Comparison-with-Publicly-Datasets.png\\\" alt=\\\"\\\" style=\\\"width: 100%; max-height: 60vh; object-fit: contain; background: #141414; border-radius: 8px\\\">\u003C/img>\\n \u003Cp class=\\\"img-text\\\" style=\\\"position: absolute; top: calc(100% + 16px); left: 0; right: 0;text-align: center; overflow: hidden; white-space: nowrap; text-overflow: ellipsis; line-height: 22px; color: #A1A1A1; font-size: 14px\\\">\\n \u003C/p>\\n\u003C/div>\\n\\nOur OmniHD-Scenes dataset contains data from **six 4D radar** **systems** acquired with high-resolution cameras and LiDAR, and also includes data from **day/night and bad-weather scenarios** to accommodate more complex working conditions. The proposed dataset also includes **segmentation and occupancy labels**, thereby fully supporting **multi-view and multi-sensor tasks**.\\n\\n## Sensor And Controller Specifications\\n\\n\u003Cdiv class=\\\"img-wrap has-caption center\\\" style=\\\"width: 100%; position: relative; margin-bottom: 62px\\\">\\n \u003Cimg src=\\\"https://global-blog.oss-ap-southeast-1.aliyuncs.com/abaka/20250325-OmniHD-Scenes/Sensor-And-Controller-Specifications.png\\\" alt=\\\"\\\" style=\\\"width: 100%; max-height: 60vh; object-fit: contain; background: #141414; border-radius: 8px\\\">\u003C/img>\\n \u003Cp class=\\\"img-text\\\" style=\\\"position: absolute; top: calc(100% + 16px); left: 0; right: 0;text-align: center; overflow: hidden; white-space: nowrap; text-overflow: ellipsis; line-height: 22px; color: #A1A1A1; font-size: 14px\\\">\\n \u003C/p>\\n\u003C/div>\\n\\n## Annotation\\n\\n\u003Cdiv class=\\\"img-wrap has-caption center\\\" style=\\\"width: 100%; position: relative; margin-bottom: 62px\\\">\\n \u003Cimg src=\\\"https://global-blog.oss-ap-southeast-1.aliyuncs.com/abaka/20250325-OmniHD-Scenes/MooreData-Platform-Annotation-Pipeline.png\\\" alt=\\\"MooreData Platform Annotation Pipeline\\\" style=\\\"width: 100%; max-height: 60vh; object-fit: contain; background: #141414; border-radius: 8px\\\">\u003C/img>\\n \u003Cp class=\\\"img-text\\\" style=\\\"position: absolute; top: calc(100% + 16px); left: 0; right: 0;text-align: center; overflow: hidden; white-space: nowrap; text-overflow: ellipsis; line-height: 22px; color: #A1A1A1; font-size: 14px\\\">\\n MooreData Platform Annotation Pipeline\\n \u003C/p>\\n\u003C/div>\\n\\nSince Tesla introduced the concept of 4D annotation,it has become a pivotal component of the data closed-loop process. This annotation technique utilizes poses to establish temporal relationships and represents traffic participants and road information over a period using dense point cloud reconstruction. Compared to traditional 3D annotation methods, the reconstructed map is denser, exhibits stronger global consistency, offers enhanced visual effects, significantly reduces repetitive tasks, and utilizes more prior information to ensure data reliability. Utilizing 4D tools for data generation can substantially lower data production costs and enhance data quality. In this study, we implemented semi-automatic 4D annotation using the MooreData platform solution, with the data being processed in clips.\\n\\n## Visualization Annotation Result\\n\\n\u003Cdiv class=\\\"img-wrap has-caption\\\" style=\\\"width: 100%\\\">\\n \u003Cvideo \\n controls\\n poster=\\\"https://global-blog.oss-ap-southeast-1.aliyuncs.com/2077ai/20241212/OmniHD-Scenes/annotation_results_isplay_rain_scene1.jpg\\\"\\n data-title=\\\"Annotation Result Display - Rain Scene\\\"\\n data-description=\\\"Annotation Result Display - Rain Scene\\\"\\n style=\\\"max-height: 60vh; width: 100%; background: #141414\\\"\\n >\\n \u003Csource src=\\\"https://global-blog.oss-ap-southeast-1.aliyuncs.com/2077ai/20241212/OmniHD-Scenes/annotation_results_isplay_rain_scene.mp4\\\" type=\\\"video/mp4\\\">\\n \u003C/video>\\n \u003Cp style=\\\"margin:8px 0 24px; text-align: center; overflow: hidden; white-space: nowrap; text-overflow: ellipsis; line-height: 22px; color: #A1A1A1; font-size: 14px\\\">Annotation Result Display - Rain Scene\u003C/p>\\n\u003C/div>\\n\\n\u003Cdiv class=\\\"img-wrap has-caption\\\" style=\\\"width: 100%;\\\">\\n \u003Cvideo \\n controls\\n poster=\\\"https://global-blog.oss-ap-southeast-1.aliyuncs.com/2077ai/20241212/OmniHD-Scenes/annotation_results_isplay_night_scene1.jpg\\\"\\n data-title=\\\"Annotation Result Display - Night Scene\\\"\\n data-description=\\\"Annotation Result Display - Night Scene\\\"\\n style=\\\"max-height: 60vh; width: 100%; background: #141414\\\"\\n >\\n \u003Csource src=\\\"https://global-blog.oss-ap-southeast-1.aliyuncs.com/2077ai/20241212/OmniHD-Scenes/annotation_results_isplay_night_scene.mp4\\\" type=\\\"video/mp4\\\">\\n \u003C/video>\\n \u003Cp style=\\\"margin:8px 0 24px; text-align: center; overflow: hidden; white-space: nowrap; text-overflow: ellipsis; line-height: 22px; color: #A1A1A1; font-size: 14px\\\">Annotation Result Display - Night Scene\u003C/p>\\n\u003C/div>\\n\\n\u003Cdiv class=\\\"img-wrap has-caption\\\" style=\\\"width: 100%\\\">\\n \u003Cvideo \\n controls\\n poster=\\\"https://global-blog.oss-ap-southeast-1.aliyuncs.com/2077ai/20241212/OmniHD-Scenes/annotation_results_isplay_day_scenario1.jpg\\\"\\n data-title=\\\"Annotation Results Display - Day Scenario\\\"\\n data-description=\\\"Annotation Results Display - Day Scenario\\\"\\n style=\\\"max-height: 60vh; width: 100%; background: #141414\\\"\\n >\\n \u003Csource src=\\\"https://global-blog.oss-ap-southeast-1.aliyuncs.com/2077ai/20241212/OmniHD-Scenes/annotation_results_isplay_day_scenario.mp4\\\" type=\\\"video/mp4\\\">\\n \u003C/video>\\n \u003Cp style=\\\"margin:8px 0 24px; text-align: center; overflow: hidden; white-space: nowrap; text-overflow: ellipsis; line-height: 22px; color: #A1A1A1; font-size: 14px\\\">Annotation Results Display - Day Scenario\u003C/p>\\n\u003C/div>\\n\\n\u003Cdiv class=\\\"img-wrap has-caption\\\" style=\\\"width: 100%\\\">\\n \u003Cvideo \\n controls\\n poster=\\\"https://global-blog.oss-ap-southeast-1.aliyuncs.com/2077ai/20241212/OmniHD-Scenes/occupancy_ground_truth1.jpg\\\"\\n data-title=\\\"Occupancy ground truth\\\"\\n data-description=\\\"Occupancy ground truth\\\"\\n style=\\\"max-height: 60vh; width: 100%; background: #141414\\\"\\n >\\n \u003Csource src=\\\"https://global-blog.oss-ap-southeast-1.aliyuncs.com/2077ai/20241212/OmniHD-Scenes/occupancy_ground_truth.mp4\\\" type=\\\"video/mp4\\\">\\n \u003C/video>\\n \u003Cp style=\\\"margin:8px 0 24px; text-align: center; overflow: hidden; white-space: nowrap; text-overflow: ellipsis; line-height: 22px; color: #A1A1A1; font-size: 14px\\\">Occupancy ground truth\u003C/p>\\n\u003C/div>\\n\\nOccupancy ground truth\\n\\n## Dataset Statistics\\n\\nThe OmniHD-Scenes dataset predominantly includes data for urban areas and overpass roads with complex traffic patterns, and spans a range of weather conditions and different times of day. Significantly, it comprises data for a substantial number of challenging scenarios, such as rainy conditions (33%) and night scenes (28%), offering valuable opportunities for the development and evaluation of more robust perception algorithms.\\n\\n\u003Cdiv class=\\\"img-wrap has-caption center\\\" style=\\\"width: 100%; position: relative; margin-bottom: 62px\\\">\\n \u003Cimg src=\\\"https://global-blog.oss-ap-southeast-1.aliyuncs.com/abaka/20250325-OmniHD-Scenes/Scene-Element-Distribution.png\\\" alt=\\\"\\\" style=\\\"width: 100%; max-height: 60vh; object-fit: contain; background: #141414; border-radius: 8px\\\">\u003C/img>\\n \u003Cp class=\\\"img-text\\\" style=\\\"position: absolute; top: calc(100% + 16px); left: 0; right: 0;text-align: center; overflow: hidden; white-space: nowrap; text-overflow: ellipsis; line-height: 22px; color: #A1A1A1; font-size: 14px\\\">\\n \u003C/p>\\n\u003C/div>\\n\\nMoreover, a notable feature of OmniHD-Scenes is its incorporation of multi-view 4D radar. Fig. 12 illustrates the point-cloud quantity distributions per frame for both the LiDAR and 4D-radar data. The LiDAR point-cloud counts per frame are concentrated between 180K and 210K points, whereas those for the 4D radar primarily range from 2K to 4K points. Therefore,in terms of point-cloud density, the 4D-radar data are conspicuously sparse. This sparseness will inspire researchers to explore more effective ways of leveraging the unique characteristics of 4D radar or to integrate it with other modalities for enhanced perception efficiency.\\n\\n\u003Cdiv class=\\\"img-wrap has-caption center\\\" style=\\\"width: 100%; position: relative; margin-bottom: 62px\\\">\\n \u003Cimg src=\\\"https://global-blog.oss-ap-southeast-1.aliyuncs.com/abaka/20250325-OmniHD-Scenes/Keyframe-PointCloud-Counts.png\\\" alt=\\\"\\\" style=\\\"width: 100%; max-height: 60vh; object-fit: contain; background: #141414; border-radius: 8px\\\">\u003C/img>\\n \u003Cp class=\\\"img-text\\\" style=\\\"position: absolute; top: calc(100% + 16px); left: 0; right: 0;text-align: center; overflow: hidden; white-space: nowrap; text-overflow: ellipsis; line-height: 22px; color: #A1A1A1; font-size: 14px\\\">\\n \u003C/p>\\n\u003C/div>\\n\\n\u003Cdiv class=\\\"img-wrap has-caption center\\\" style=\\\"width: 100%; position: relative; margin-bottom: 62px\\\">\\n \u003Cimg src=\\\"https://global-blog.oss-ap-southeast-1.aliyuncs.com/abaka/20250325-OmniHD-Scenes/Comparison-of-3D-Object-Detection.png\\\" alt=\\\"3D OD Model Performance\\\" style=\\\"width: 100%; max-height: 60vh; object-fit: contain; background: #141414; border-radius: 8px\\\">\u003C/img>\\n \u003Cp class=\\\"img-text\\\" style=\\\"position: absolute; top: calc(100% + 16px); left: 0; right: 0;text-align: center; overflow: hidden; white-space: nowrap; text-overflow: ellipsis; line-height: 22px; color: #A1A1A1; font-size: 14px\\\">\\n 3D OD Model Performance\\n \u003C/p>\\n\u003C/div>\\n\\n\u003Cdiv class=\\\"img-wrap has-caption center\\\" style=\\\"width: 100%; position: relative; margin-bottom: 62px\\\">\\n \u003Cimg src=\\\"https://global-blog.oss-ap-southeast-1.aliyuncs.com/abaka/20250325-OmniHD-Scenes/OCC-Model-Performance.png\\\" alt=\\\"OCC Model Performance\\\" style=\\\"width: 100%; max-height: 60vh; object-fit: contain; background: #141414; border-radius: 8px\\\">\u003C/img>\\n \u003Cp class=\\\"img-text\\\" style=\\\"position: absolute; top: calc(100% + 16px); left: 0; right: 0;text-align: center; overflow: hidden; white-space: nowrap; text-overflow: ellipsis; line-height: 22px; color: #A1A1A1; font-size: 14px\\\">\\n OCC Model Performance\\n \u003C/p>\\n\u003C/div>\\n\\n## Conclusion And Future Work\\n\\nIn the future, we plan to expand OmniHD-Scenes to facilitate additional tasks and benchmarks, including trajectory prediction, visual grounding, and end-to-end autonomous driving.\\n\\n## ACKNOWLEDGEMENT\\n\\nThanks to Intelligent Connected Vehicle inspection Center (Hunan) of CAERI Co., Ltd and Shanghai Geometrical Perception and Learning Co., Ltd.\\n\\n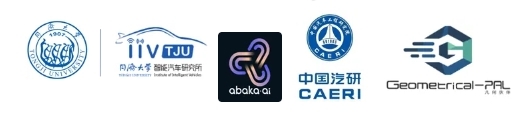\\n\",\"headings\":[{\"level\":2,\"text\":\"Overview\",\"children\":[]},{\"level\":2,\"text\":\"Abstract\",\"children\":[]},{\"level\":2,\"text\":\"Overview\",\"children\":[]},{\"level\":2,\"text\":\"Sensor And Controller Specifications\",\"children\":[]},{\"level\":2,\"text\":\"Annotation\",\"children\":[]},{\"level\":2,\"text\":\"Visualization Annotation Result\",\"children\":[]},{\"level\":2,\"text\":\"Dataset Statistics\",\"children\":[]},{\"level\":2,\"text\":\"Conclusion And Future Work\",\"children\":[]},{\"level\":2,\"text\":\"ACKNOWLEDGEMENT\",\"children\":[]}]},{\"slug\":\"pin-dataset\",\"link\":\"/blog/pin-dataset\",\"title\":\"PIN Dataset: A Unified Paradigm for Multimodal Learning\",\"desc\":\"A Unified Paradigm for Multimodal Learning\",\"bannerImg\":\"/bg3.png\",\"date\":\"2024-09-12\",\"content\":\"# PIN Dataset: A Unified Paradigm for Multimodal Learning\\n2077AI Foundation is proud to introduce our new project, the `PIN Multimodal Document Dataset`. This initiative emerges from our in-depth analysis of the current developmental bottlenecks in multimodal large models, aiming to catalyze exponential growth in multimodal AI through innovative data formatting.\\n\\n\u003Cdiv class=\\\"img-wrap has-caption\\\" style=\\\"width: 100%\\\">\\n \u003Cimg src=\\\"https://global-blog.oss-ap-southeast-1.aliyuncs.com/2077ai/20240912/PINDataset/image.png\\\" alt=\\\"General statisitcs of our PIN-14M dataset.\\\" style=\\\"width: 100%\\\">\\n \u003Cp style=\\\"text-align: center\\\">General statisitcs of our PIN-14M dataset.\u003C/p>\\n\u003C/div>\\n\\n**PIN: A Unifying Paradigm for Multimodal Learning**\\n\\nThe core philosophy behind the PIN project is to establish a data format that unifies multimodal learning processes and patterns. We've observed that while the text domain has solidified a \\\"text-in, text-out\\\" paradigm, the multimodal realm still lacks a cohesive, efficient training methodology. The PIN format is designed to bridge this gap, offering a more intuitive approach to blending image and text data training.\\n\\n\u003Cdiv class=\\\"img-wrap has-caption\\\" style=\\\"width: 100%\\\">\\n \u003Cimg src=\\\"https://global-blog.oss-ap-southeast-1.aliyuncs.com/2077ai/20240912/PINDataset/image1.png\\\" alt=\\\"\\nComparative analysis of traditional multimodal formats versus the proposed PIN format. The PIN format preserves rich knowledge attributes (e.g., bolding, highlighting, code), supports semantic interplay between images and text in markdown files, and enhances knowledge representation through an overall image.\\\" style=\\\"width: 100%\\\">\\n \u003Cp style=\\\"text-align: center\\\">\\nComparative analysis of traditional multimodal formats versus the proposed PIN format. The PIN format preserves rich knowledge attributes (e.g., bolding, highlighting, code), supports semantic interplay between images and text in markdown files, and enhances knowledge representation through an overall image.\u003C/p>\\n\u003C/div>\\n\\n**Innovative Data Architecture**\\n\\nPIN introduces a unique \\\"holistic pairing with interwoven content\\\" structure:\\n\\n- Holistic Pairing: Each sample comprises a Markdown file coupled with a corresponding comprehensive image.\\n- Interwoven Content: The Markdown file encapsulates text and embedded images that are intricately linked to the comprehensive image.\\n\\nThis architecture addresses the limitations of current image-text pair formats, such as overly concise captions or low relevance issues.\\n\\nMarkdown: The Optimal Knowledge Vehicle\\n\\n- Facilitates detailed articulation of knowledge attributes\\n- Supports multi-modal embedding (text, images, code, etc.)\\n- Preserves document structural integrity\\n\\nComprehensive Image: Dense Visual Knowledge Representation\\n\\n- Encapsulates rich visual data, including page layouts and design elements\\n- Excels in representing complex visual constructs like code blocks and diagrams\\n\\n\u003Cdiv class=\\\"img-wrap has-caption\\\" style=\\\"width: 100%\\\">\\n \u003Cimg src=\\\"https://global-blog.oss-ap-southeast-1.aliyuncs.com/2077ai/20240912/PINDataset/image2.png\\\" alt=\\\"\\nSamples from various subsets of the PIN-14M dataset. For each subset, one entry is extracted, showcasing both its markdown file section and the corresponding overall image.\\\" style=\\\"width: 100%\\\">\\n \u003Cp style=\\\"text-align: center\\\">\\nSamples from various subsets of the PIN-14M dataset. For each subset, one entry is extracted, showcasing both its markdown file section and the corresponding overall image.\u003C/p>\\n\u003C/div>\\n\\n**Pioneering a Unified Format for the Future**\\n\\nPIN is not merely a novel data format; it's the genesis of a unified standard for multimodal data. We envision PIN as a blueprint for future dataset creation, encompassing:\\n\\n- Conceptual Framework: Detailed exposition of the dataset's design philosophy and objectives\\n\\n- Procedural Transparency: Full disclosure of data processing workflows\\n\\n- Quality Metrics: Integration of reliable quality indicators within the data\\n\\n\u003Cdiv class=\\\"img-wrap has-caption\\\" style=\\\"width: 100%\\\">\\n \u003Cimg src=\\\"https://global-blog.oss-ap-southeast-1.aliyuncs.com/2077ai/20240912/PINDataset/image3.png\\\" alt=\\\"The overview of our process workflow.\\\" style=\\\"width: 100%\\\">\\n \u003Cp style=\\\"text-align: center\\\">The overview of our process workflow.\u003C/p>\\n\u003C/div>\\n\\n\u003Cdiv class=\\\"img-wrap has-caption\\\" style=\\\"width: 100%\\\">\\n \u003Cimg src=\\\"https://global-blog.oss-ap-southeast-1.aliyuncs.com/2077ai/20240912/PINDataset/image4.png\\\" alt=\\\"The file tree structure of an example dataset in PIN format.\\\" style=\\\"width: 100%\\\">\\n \u003Cp style=\\\"text-align: center\\\">The file tree structure of an example dataset in PIN format.\u003C/p>\\n\u003C/div>\\n\\n\u003Cdiv class=\\\"img-wrap has-caption\\\" style=\\\"width: 100%\\\">\\n \u003Cimg src=\\\"https://global-blog.oss-ap-southeast-1.aliyuncs.com/2077ai/20240912/PINDataset/image5.png\\\" alt=\\\"An example data sample of JSONL files.\\\" style=\\\"width: 100%\\\">\\n \u003Cp style=\\\"text-align: center\\\">An example data sample of JSONL files.\u003C/p>\\n\u003C/div>\\n\\n**Conclusion**\\n\\nWe have already released initial data sets. Our next iteration aims to expand the data scale to 100M while open-sourcing training models to foster community research.\\n\\nAlthough comprehensive training experiments are pending due to time and resource constraints, the PIN format opens avenues for several innovative training methodologies, including:\\n\\n- Markdown-based prediction of comprehensive images\\n\\n- Extraction of structured textual knowledge from comprehensive images\\n\\n- Multi-task learning: Concurrent training in text comprehension, image understanding, and cross-modal reasoning\\n\\nThe PIN project represents a significant stride towards our vision of a more intuitive, unified, and robust learning paradigm in multimodal AI. By addressing the fundamental challenge of data formatting, we believe PIN will pave the way for transformative advancements in multimodal large models. While there are still many areas to explore, we are confident that collaborative efforts within the community will accelerate progress, ultimately leading to a quantum leap in multimodal AI capabilities.\\n\\nWe invite the global community of researchers and developers to join us in exploring the full potential of the PIN format and pushing the boundaries of multimodal AI. Together, let's shape the future of this exciting new era in artificial intelligence.\\n\\n**Commitment to Open Source**\\n\\nAdhering to the principles of openness and sharing, we have not only open-sourced all the data but also made public the code for all processing pipelines, including filtering, deduplication, document conversion, and all other procedures. This allows researchers and developers to delve into every step of data processing and even adjust and optimize according to their needs.\\n\\n**Join Us in Shaping the Future of AI**\\n\\nThe launch of the Matrix Dataset marks a new milestone in AI pre-training data. We cordially invite AI researchers and developers worldwide to join us in leveraging this rich resource, pushing the boundaries of AI technology, and collectively shaping the future of AI.\\n\",\"headings\":[]}]",mdc:{components:{prose:true,map:{}},headings:{anchorLinks:{h1:false,h2:true,h3:true,h4:true,h5:false,h6:false}}},"seo-utils":{canonicalQueryWhitelist:["page","sort","filter","search","q","category","tag"]}},app:{baseURL:"/",buildId:"b5e3f67d-2c73-49d9-97ad-042c03f5f6c1",buildAssetsDir:"/_nuxt/",cdnURL:""}}